Jan K- nearest neighbors is a classification (or regression) algorithm that in order to determine. You can build centroids (as in k - means ) based on your labeled data.
What are the main similiarities between K - means and K. SepUsing real data means as centroids for clustering - Cross. AprHow to take the index of the nearest centroid as a feature.
FebMorefrom stats. How is the k-nearest neighbor algorithm different from k. How-is-the-k-nearest-neighbor-algor. Hi We will start with understanding how k-NN, and k - means clustering works. Applying the 1- nearest neighbor classifier to the cluster centers obtained by k - means classifies new data into the existing clusters.
This is known as nearest. In machine learning, a nearest centroid classifier or nearest prototype classifier is a classification model that assigns to observations the label of the class of training samples whose mean (centroid) is closest to the observation.
Nearest_centroid_classifieren. Thus, K - means clustering represents an unsupervised algorithm, mainly used for clustering, while KNN is a supervised learning algorithm used for classification. K - means clustering uses “centroids”, K different randomly-initiated points in the data, and assigns every data point to the nearest centroid. After every point has.
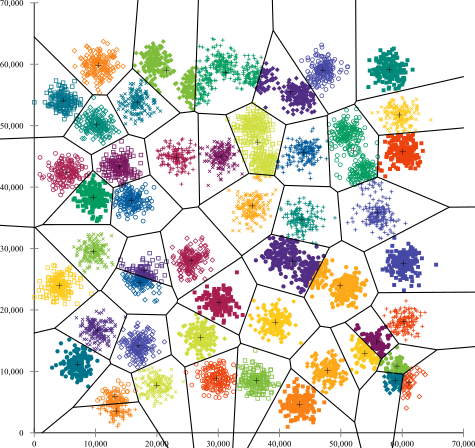
The classsklearn. Calculate the centroid or mean of all objects in each cluster. Return the mean accuracy on the given test data and labels. NN) is a very useful and easy-implementing.

Dec Select k centroids. Reassign centroid value to be the. Assign data points to nearest centroid. K - Means is a clustering algorithm with a wide range of applications in data.
Sep In addition, a harmonic mean distance metric was introduced in the multi-local means -based k -harmonic nearest neighbor (MLMKHNN) classifier. Aug Each new case is assigned to the cluster with the nearest centroid. In classification problems, lots of methods devote. K - means starts by selecting k random data points as the initial set of centroids, which is then.
For each point, we maintain pointer to its nearest centroid. K - nearest neighbor (KNN) rule is a simple and effective algorithm in pattern classification. In this article, we propose a local. Non-parametric mode finding: density estimation.
Hierarchical clustering. Jan Computing the distances between all data points and the existing K centroids and re-assigning each data point to its nearest centroid. Cluster evaluation. Nov K - Means begins with k randomly placed centroids.
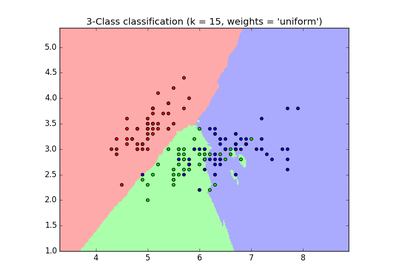
Finds the nearest centroid for. Once we have initialized the centroids, we assign each point to the closest cluster. K - means is an algorithm that is great for finding clusters in many types of datasets.
All the points nearest each of.
No comments:
Post a Comment
Note: only a member of this blog may post a comment.