Apr If you use MiniBatch k - means, it will not converge, so you need one of the other criteria. The usual one is the number of iterations. FebWhat stop - criteria for agglomerative hierarchical clustering are.
DecMorefrom stats. K-Means Clustering: From A to Z. Everything you need to. Sep The stopping criterion tells our algo when to stop updating the clusters.
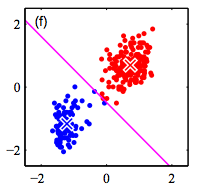
It is important to note that setting a stopping criterion would not necessarily. May Setting no change as a stopping criteria is a bad idea. S Ullman - Cited by - Related articles k-means clustering - en. K-means_clusteringen.
K - means convergence ( stopping ) criterion. Using a different distance function other than (squared) Euclidean distance may stop the algorithm from converging. The most common criterion for definining clusters is the k - means criterion.
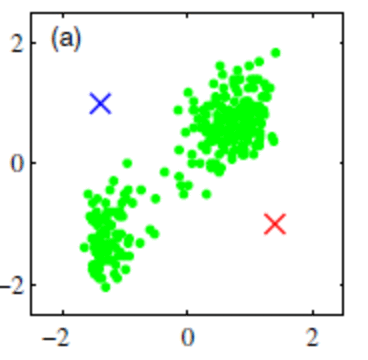
Repeat step and until convergence to some stopping criterion. Jan define an initial set of K centroids, C. Step 2: C = WeightedLloyd(P, C, K ). Stopping Criterion do. Step 3: Construct a dataset partition P. Aug Learn how to set the stopping criteria for your k - Means algorithm in the H2O machine learning library using R, Python, Java, or Scala. Jul Finally, a stopping criterion is verified.
Sep Theoretically, k - means should terminate when no more pixels are. In software, we typically terminate when one of the following criteria is met.

These two steps are then repeated until some stopping criterion are reached. A stopping criteria is evaluated to determine if further data should be accessed.
One of the most well-known algorithms for clustering is the K - Means algorithm. However, it seems advisable. In practice, we often stop when the decrease in the objective function.
It has flags as below: cv. TERM_CRITERIA_EPS - stop the algorithm iteration if specified accuracy, epsilon, is reached. Runs the k - means clustering algorithm, with optional new data. Kmeans which is a method.
This study proposes RCE. Options for controlling the iterative algorithm for minimizing the fitting criteria. AWS rstudio-pubs-static. In k - means clustering, each cluster is represented by its center (i.e, centroid).
Ames data using k - means clustering and the elbow criterion. If the stopping criteria achieved.
No comments:
Post a Comment
Note: only a member of this blog may post a comment.